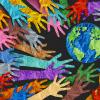
In recent years, crowdsourcing, which involves recruiting members of the public to help collect data, has been tremendously helpful to provide researchers with unique and rich datasets, while also engaging the public in the process of scientific discovery. In a new study, an international team of researchers explored how crowdsourcing projects can make the most effective use of volunteer contributions.
Data collection activities through crowdsourcing range from field-based activities, such as bird watching, to online activities such as image classification for projects like the highly successful Galaxy Zoo, in which participants classify galaxy shapes, and Geo-Wiki, where satellite images are interpreted for land cover, land use, and socioeconomic indicators. Getting input from so many participants analyzing a set of images, however, raises questions around how accurate the submitted responses actually are. While there are methods to ensure the accuracy of data gathered in this way, they often have implications for crowdsourcing activities such as sampling design and associated costs.
In their study just published in the journal PLOS ONE, researchers from IIASA and international colleagues explored the question of accuracy by investigating how many ratings of a task need to be completed before researchers can be reasonably certain of the correct answer.
“Many types of research with public participation involve getting volunteers to classify images that are difficult for computers to distinguish in an automated way. However, when a task has to be repeated by many people, it makes the assignment of tasks to the people performing them more efficient if you are certain about the correct answer. This means less time of volunteers or paid raters is wasted, and scientists or others requesting the tasks can get more from the limited resources available to them,” explains Carl Salk, an alumnus of the IIASA Young Scientists Summer Program (YSSP) and long-time IIASA collaborator currently associated with the Swedish University of Agricultural Sciences.
The researchers developed a system for estimating the probability that the majority response to a task is wrong, and then stopped assigning the task to new volunteers when that probability became sufficiently low, or the probability of ever getting a clear answer became low. They demonstrated this process using a set of over 4.5 million unique classifications by 2,783 volunteers of over 190,000 images assessed for the presence or absence of cropland. The authors point out that, had their system been implemented in the original data collection campaign, it would have eliminated the need for 59.4% of volunteer ratings, and that if the effort had been applied to new tasks, it would have allowed more than double the amount of images to be classified with the same amount of labor. This shows just how effective this method can be in making more efficient use of limited volunteer contributions.
According to the researchers, this method can be applied to nearly any situation where a yes or no (binary) classification is required, and the answer may not be highly obvious. Examples could include classifying other types of land use, for instance: “is there forest in this picture?”; identifying species, by asking: “is there a bird in this picture?”; or even the sort of 'ReCaptcha' tasks that we do to convince websites that we are human, such as: “is there a stop light in this picture?”. The work can also contribute to better answering questions that are important to policymakers, such as how much land in the world is used for growing crops.
“As data scientists turn increasingly to machine learning techniques for image classification, the use of crowdsourcing to build image libraries for training continues to gain importance. This study describes how to optimize the use of the crowd for this purpose, giving clear guidance when to refocus the efforts when either the necessary confidence level is reached or a particular image is too difficult to classify,” concludes study coauthor, Ian McCallum, who leads the Novel Data Ecosystems for Sustainability Research Group at IIASA.
Reference
Salk, C., Moltchanova, E., See, L., Sturn, T., McCallum, I., Fritz, S. (2022). How many people need to classify the same image? A method for optimizing volunteer contributions in binary geographical classifications. PLOS ONE DOI: 10.1371/journal.pone.0267114
News

22 July 2024
Are sustainable aviation fuels truly sustainable?
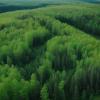
17 July 2024
Forests endure as carbon sink despite regional pressures

16 July 2024